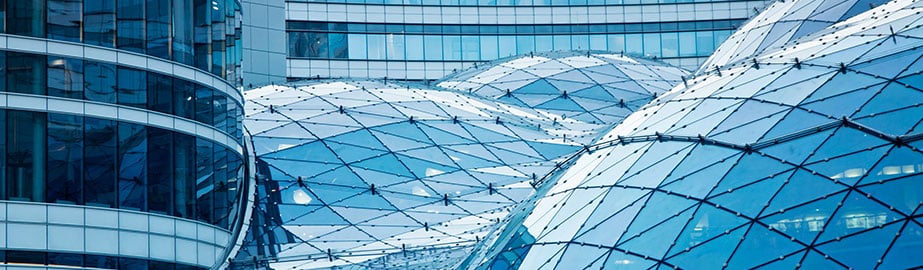
Front office portfolio analytics engines produce powerful ex-post and ex-ante performance and risk analytics that require precise and accurate data to yield meaningful results. We’ve all heard it before: data is the backbone of any successful implementation. In any system, if you put garbage in, you get garbage out. This is especially true for front office portfolio analytics implementations where precision of results is often driven by data quality and timeliness. However, data isn’t the only story here—results are also significantly impacted by the decision of whether to implement holdings-based or transaction-based models. When evaluating which path to take, organizations must understand the level of the complexity of each path and the impact not only on implementations but on the ongoing support model.
Holdings and Transaction Precision Considerations
A holdings feed provides daily views of positions that go beyond just the end of day price and quantity of the positions held. Classifications such as asset type and sector can accomplish custom groupings of assets in dashboards and outputs. Position weights and performance calculation accuracy increases with position detail such as principal, income, and accruals. Further precision can be built for fixed income positions by defining measures such as various yield to maturities, effective duration, and convexity. Lastly, to achieve a holdings level gain/loss view, a holdings file can even contain the average cost per share of the positions held.
Transactions introduce a secondary level of precision whose benefits really boil down to accuracy of performance and ability to run security level profit and loss reporting. For example, instead of coming up with a gain/loss on a position using cost averages, transactions provide the actual execution price for each lot of a security purchased and sold. This allows for the calculation of the exact gain/loss on the trade. To provide further precision on a trade, transactional portfolio analytics data can capture the actual sale and purchase accrual for the interest paid or received at the time of the transaction. Tracking dividend accruals and payments for equities is more precise given the more granular nature of transactions vs holdings data files. Another benefit with transaction data over holdings data is that cash movements can be traced to buys, sells, income, and interest payments intraday. This is the principal reason that transaction files typically capture trade date cash instead of settlement date cash that is classically captured in a holdings file.
These transactional data benefits allow for more powerful and precise ex-post analytics and attribution which can help improve the investment decision making process. While holdings are a point in time snapshot of a portfolio’s positions, transactions provide more detail into when those positions were bought or sold, the order fulfillment price, and can even trace the lot of the position sold to capture the true gain or loss on the transaction.
Technical Challenges: Holdings vs Transactions
In either instance, the configurations of these types of platforms are highly complex and can be customized to meet the specific needs around portfolio analytics and the nuances of various strategies and security types held. This translates into how to present data into these systems that requires an advanced level of design and analysis. The source of the data, whether it is holdings or transactions, is an important consideration as well. Drastically different results can be achieved using custody data vs ABOR data vs IBOR data. The same is true for the frequency of the data being provided. Analytics can show more meaningful results for data being provided into these tools daily vs weekly or monthly and even intraday vs daily.
While the above is true for both holdings and transactions, implementing a transaction file is more time consuming and taxing on the asset manager. Systems that support transaction file data sources may ask the asset manager to compile net activity by security and map transaction data to holdings data to work properly. Also, to get transactions to work appropriately, the various transaction types from the source system need to be mapped to the appropriate buckets in the portfolio analytics system. While netting and mapping transactions is a simple concept, the reality is that the marriage isn’t always apples to apples and requires significantly complex and custom integration logic to power the analytics system. The burden to complete this work is placed on the asset manager providing the data to the vendor system.
That said, there have been recent advancements in the layout and implementation requirements for transaction-based data feeds. System vendors are moving toward integration as a service where they would map raw data into their systems through vendor supported transaction data import logic. The movement towards intraday transactions has opened the door for order management systems (OMS) system integration. Since the OMS is the originating system for each transaction, this makes it the optimal system to quickly provide intraday transactions to the portfolio analytics system. Given that most OMS deployments lack critical accounting transactions like accruals and corporate actions, front office vendors need to bridge this gap. If done properly, the OMS raw data feeds would not only simplify the data format requirements, but provide the flexibility to achieve intraday or near real-time syncing of transaction data into the analytics solution. There are some applications that have come further than others on this front, but these implementations require the vendor to have an exhaustive understanding of the asset manager’s OMS transaction types as well as their accounting methodologies.
The Bottom Line
Understanding the implementation and support requirements for holdings- or transactions-based portfolio analytics implementations is critical to ensuring a successful deployment and support model. There are many benefits and complexities that need to be taken into consideration when making this decision including data governance and technical and operational support models. Chasing precision introduces an underappreciated level of complexity in terms of the data integration and ongoing support model. In determining which path to choose, whether it’s holdings or transactions, one must evaluate the chase for precision against the cost to achieve and maintain it.
Comments